A paradigm shift in balance sheet management
Insights for financial and risk management professionals following CeFPro's Balance Sheet Management event.
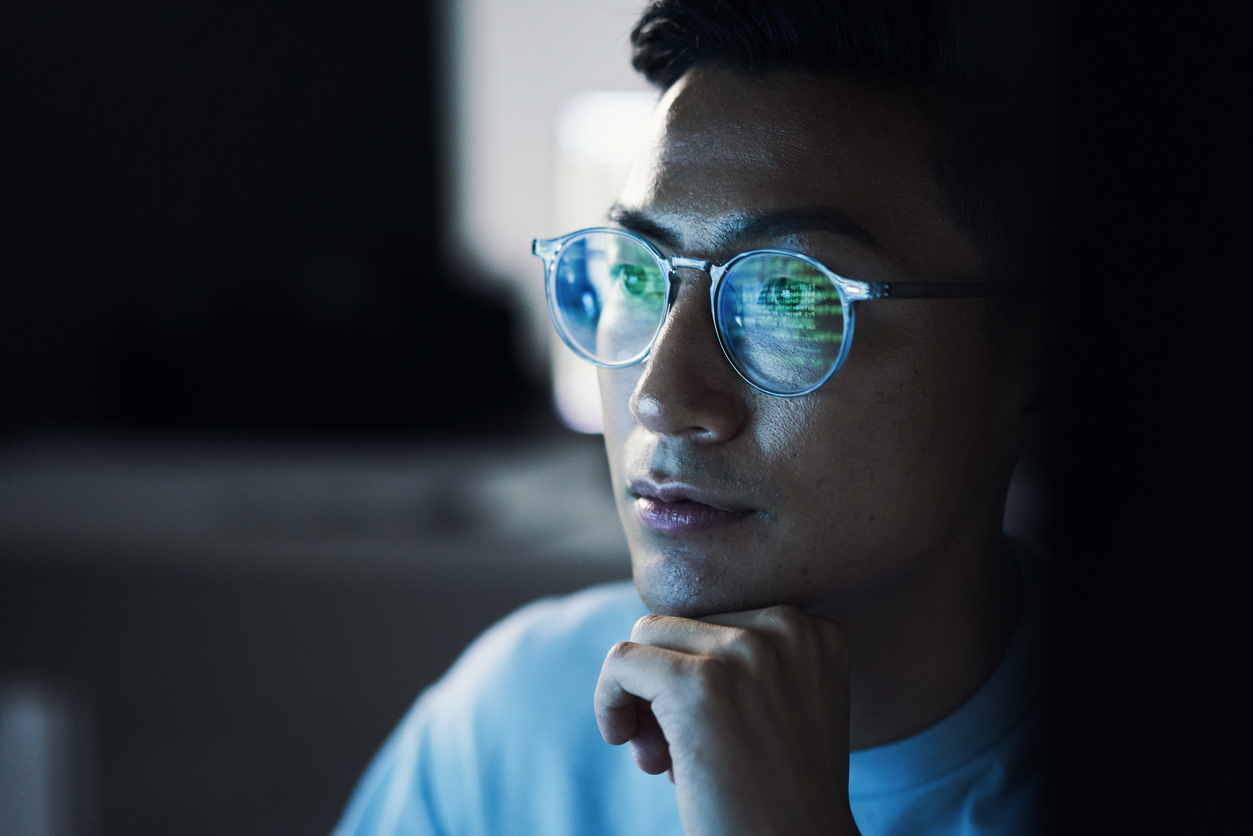
In the wake of the 2023 banking crisis, treasury, asset-liability management (ALM), and risk practitioners convened at the CeFPro Balance Sheet Management conference to reflect on the tumultuous events and discuss strategies for better anticipating and responding to future crises. This article provides insights for financial and risk management professionals following the event.
The challenges surrounding balance sheet management
Business Context: Recent Past and Perspectives
The past year has been challenging for the banking sector. The Federal Reserve Board's aggressive interest rate hikes since March 2022, aimed at taming persistent inflation, precipitated a significant regional banking crisis. This response, while necessary, brought about an overwhelming regulatory reaction and substantial capital costs. The gradual slowing of inflation, evidenced by the latest October figures, suggests that the Fed might be nearing the end of its hiking cycle. While the main economic scenario is a soft landing, a recession could still happen, especially in sectors like commercial real estate (CRE), which faces daunting refinancing challenges in 2024 and retail credit. The ripple effects on banks could be significant, casting a shadow over the outlook for 2024.
Lessons Learned & Critical Improvement Questions
Interest Rate Hedges and Cooperative Responses
As interest rates soared, most regional banks turned early in 2022 to expensive but effective interest rate hedges to protect their securities investments – a strategy contrasting sharply with the approach of Silicon Valley Bank (SVB) and its substantial losses on available-for-sale (AFS) securities, that came along with an opposite hedges’ reduction. The crisis's peak in March 2023 highlighted the value of local Federal Home Loan Banks' (FHLBs) cooperation. However, concerns were raised about the readiness of all local U.S. banks, not all of which have accounts with FHLBs.
Modeling and Simulation Capabilities
The crisis underscored the need for enhanced modeling and simulation capabilities to better anticipate future challenges. Key areas of focus include:
1. Business Modeling:
- Modeling deposit behaviors and resources, considering the rapid flight to quality and its costly impact on funding alternatives.
- Integrating stress tests across risk silos (liquidity, credit, and market) using comprehensive scenarios.
2. Technical Capabilities:
- Developing real-time analysis capabilities to match the pace of crises.
- Establishing best practices for model management.
- Integrating extensive historical data series for rates and inflation.
3. Regulatory Framework:
- Adapting to Basel’s Endgame, which moves away from advanced approaches for credit risk and introduces a new market risk framework affecting banking book transactions.
- Transitioning model risk management to include AI models for the aforementioned modeling challenges.
These discussions are crucial for preparing the banking sector for future uncertainties. By learning from the past and innovating in risk management and modeling techniques, financial institutions can fortify themselves against the complexities of an ever-evolving economic landscape.
Embracing Modern Solutions: Data Mesh at the Fore
In light of these challenges, particularly the pressing need for real-time responses, granular data analysis, and comprehensive scenario planning, the principles of Data Mesh have emerged as a highly relevant solution since 2018. This approach directly addresses the deep-seated issues of organizational and data silos that significantly impede effective balance sheet management.
Data Mesh advocates for a decentralized approach to data management, placing the ownership and responsibility of data in the hands of those closest to its context – the individual business units like ALM, Treasury, and Risk Management. By breaking down silos, this method ensures that relevant and up-to-date data is readily available, enabling faster and more accurate responses to rapidly evolving market conditions, such as those seen in the case of Silicon Valley Bank.
Furthermore, Data Mesh fosters a culture where data is treated as a product, enhancing its quality, usability, and relevance. This principle is crucial in addressing the data dilemma where granular insights into customer profiles and market segments are needed for robust risk assessment and scenario analysis. The approach also aligns with the shift towards proactive 'what could happen' risk management strategies, equipping financial institutions with the agility to navigate uncharted waters and anticipate potential credit risks.
In essence, Data Mesh as a set of principles offers a transformative path forward, enabling financial institutions to overcome the traditional constraints of data governance and analytics, and emerge more resilient in the face of unprecedented economic challenges.
Leaner and More Effective Risk Analytics
Effective risk analytics could serve as a panacea for staffing challenges, especially beneficial for medium and small banks that may not have the resources to scale up rapidly in response to economic headwinds.
Promoting Self-Service Analytics and Data Ownership
The paradigm shift towards self-service analytics and data ownership is pivotal. The data mesh concept advocates for a decentralized approach to data management, encouraging domain-level ownership and governance. This shift aligns perfectly with the current needs of front office, middle office, and back office professionals.
For front-officers and risk officers, real-time access to integrated data means being able to make quick, informed decisions that could mitigate or capitalize on market movements.
For risk managers, it means having the tools to predict and model various risk scenarios accurately.
And for those in supporting functions, it means ensuring that the data governance framework is robust, reliable, and responsive to the needs of the entire bank.
By embedding analytics and decision-making tools within the domain of the professionals who use them, banks can become more agile. Each domain can own and quality-control their data, leading to more accurate risk models, predictions, and ultimately, decision-making.
The ongoing economic volatility serves as a catalyst for financial institutions to reassess their balance sheet management strategies. The adoption of self-service analytics and data ownership, in accordance with data mesh principles, stands out not just as a modern convenience, but as a strategic imperative.
By decentralizing analytics and encouraging domain-level data governance, banks can foster a culture of quick response, resilience, and adaptability that will be crucial in navigating the uncertain waters of today's financial landscape.
To learn more, contact us at: https://opensee.io/contact